Statistical Characterization of the Morphologies of Nanoparticles through Machine Learning Based Electron Microscopy Image Analysis
페이지 정보

조회 2,235회 작성일 20-12-19 22:02
본문
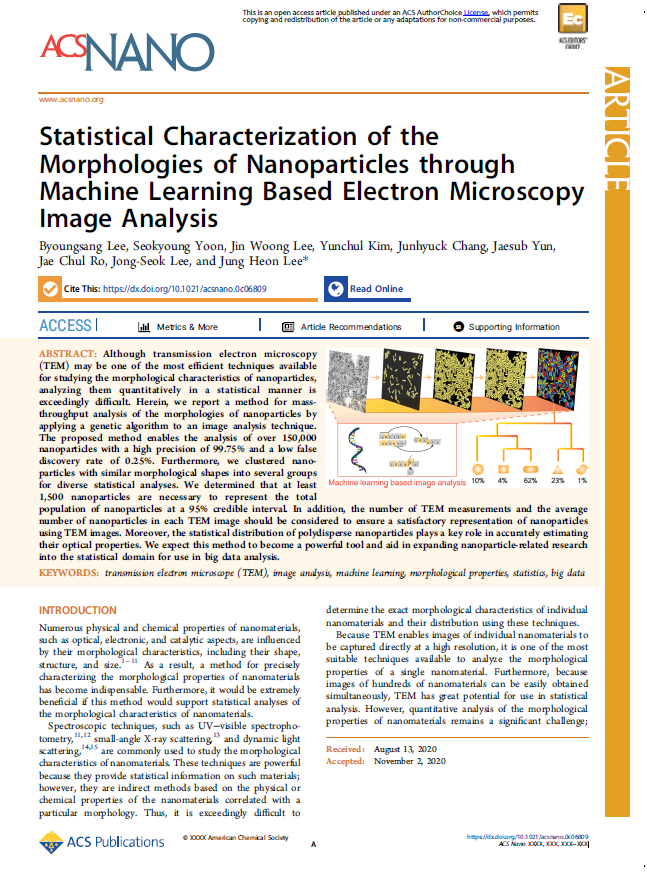
Journal | ACS Nano, 2020, ASAP (Open access via ACS Editors' Choice) |
---|---|
Name | Lee, B., Yoon, S., Lee, J. W., Kim, Y., Chang, J., Yun, J., Ro, J. C., Lee, J.-S. and Lee, J. H. |
Year | 2020 |
Although transmission electron microscopy (TEM) may be one of the most efficient techniques available for studying the morphological characteristics of nanoparticles, analyzing them quantitatively in a statistical manner is exceedingly difficult. Herein, we report a method for mass-throughput analysis of the morphologies of nanoparticles by applying a genetic algorithm to an image analysis technique. The proposed method enables the analysis of over 150,000 nanoparticles with a high precision of 99.75% and a low false discovery rate of 0.25%. Furthermore, we clustered nanoparticles with similar morphological shapes into several groups for diverse statistical analyses. We determined that at least 1,500 nanoparticles are necessary to represent the total population of nanoparticles at a 95% credible interval. In addition, the number of TEM measurements and the average number of nanoparticles in each TEM image should be considered to ensure a satisfactory representation of nanoparticles using TEM images. Moreover, the statistical distribution of polydisperse nanoparticles plays a key role in accurately estimating their optical properties. We expect this method to become a powerful tool and aid in expanding nanoparticle-related research into the statistical domain for use in big data analysis.