Machine learning predictive model based on national data for fatal accidents of construction workers.
페이지 정보

조회 2,219회 작성일 20-12-19 21:56
본문
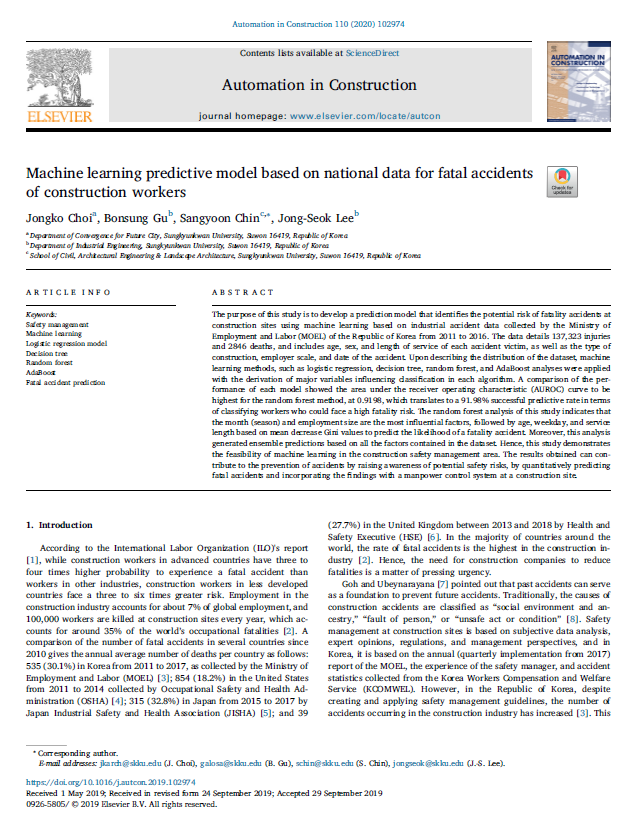
Journal | Automation in Construction, 110, 102974. |
---|---|
Name | Choi, J., Gu, B., Chin, S., and Lee, J.-S. |
Year | 2020 |
The purpose of this study is to develop a prediction model that identifies the potential risk of fatality accidents at construction sites using machine learning based on industrial accident data collected by the Ministry of Employment and Labor (MOEL) of the Republic of Korea from 2011 to 2016. The data details 137,323 injuries and 2846 deaths, and includes age, sex, and length of service of each accident victim, as well as the type of construction, employer scale, and date of the accident. Upon describing the distribution of the dataset, machine learning methods, such as logistic regression, decision tree, random forest, and AdaBoost analyses were applied with the derivation of major variables influencing classification in each algorithm. A comparison of the performance of each model showed the area under the receiver operating characteristic (AUROC) curve to be highest for the random forest method, at 0.9198, which translates to a 91.98% successful predictive rate in terms of classifying workers who could face a high fatality risk. The random forest analysis of this study indicates that the month (season) and employment size are the most influential factors, followed by age, weekday, and service length based on mean decrease Gini values to predict the likelihood of a fatality accident. Moreover, this analysis generated ensemble predictions based on all the factors contained in the dataset. Hence, this study demonstrates the feasibility of machine learning in the construction safety management area. The results obtained can contribute to the prevention of accidents by raising awareness of potential safety risks, by quantitatively predicting fatal accidents and incorporating the findings with a manpower control system at a construction site.