A Learning Objective Controllable Sphere-Based Method for Balanced and Imbalanced Data Classification
페이지 정보

조회 1,305회 작성일 21-12-06 17:50
본문
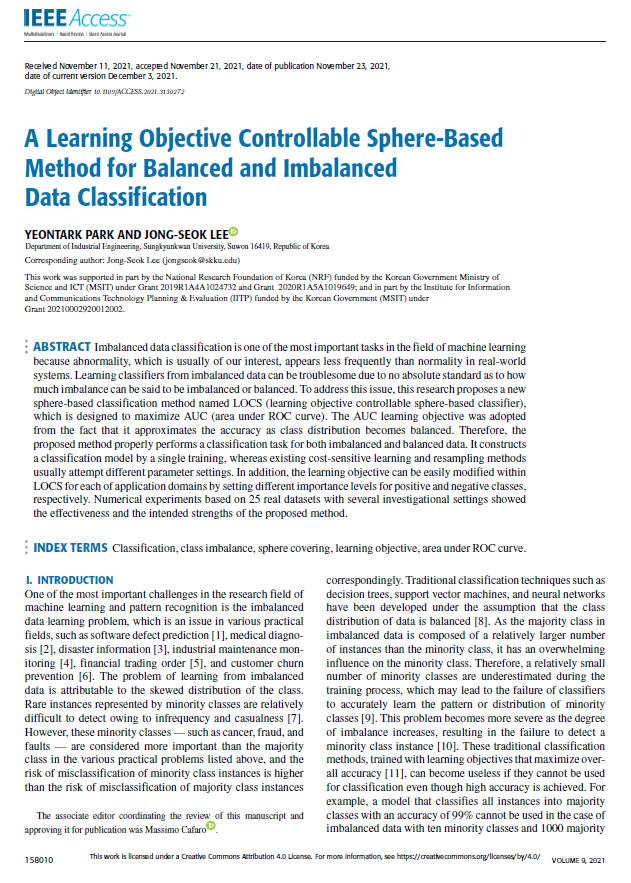
Journal | IEEE Access, 9, 158010 - 158026. |
---|---|
Name | Y. Park and J.-S. Lee |
Year | 2021 |
Imbalanced data classification is one of the most important tasks in the field of machine learning because abnormality, which is usually of our interest, appears less frequently than normality in real-world systems. Learning classifiers from imbalanced data can be troublesome due to no absolute standard as to how much imbalance can be said to be imbalanced or balanced. To address this issue, this research proposes a new sphere-based classification method named LOCS (learning objective controllable sphere-based classifier), which is designed to maximize AUC (area under ROC curve). The AUC learning objective was adopted from the fact that it approximates the accuracy as class distribution becomes balanced. Therefore, the proposed method properly performs a classification task for both imbalanced and balanced data. It constructs a classification model by a single training, whereas existing cost-sensitive learning and resampling methods usually attempt different parameter settings. In addition, the learning objective can be easily modified within LOCS for each of application domains by setting different importance levels for positive and negative classes, respectively. Numerical experiments based on 25 real datasets with several investigational settings showed the effectiveness and the intended strengths of the proposed method.