Gently Sloped and Extended Classification Margin for Overconfidence Relaxation of Out-of-Distribution Samples
페이지 정보

조회 274회 작성일 25-02-13 10:42
본문
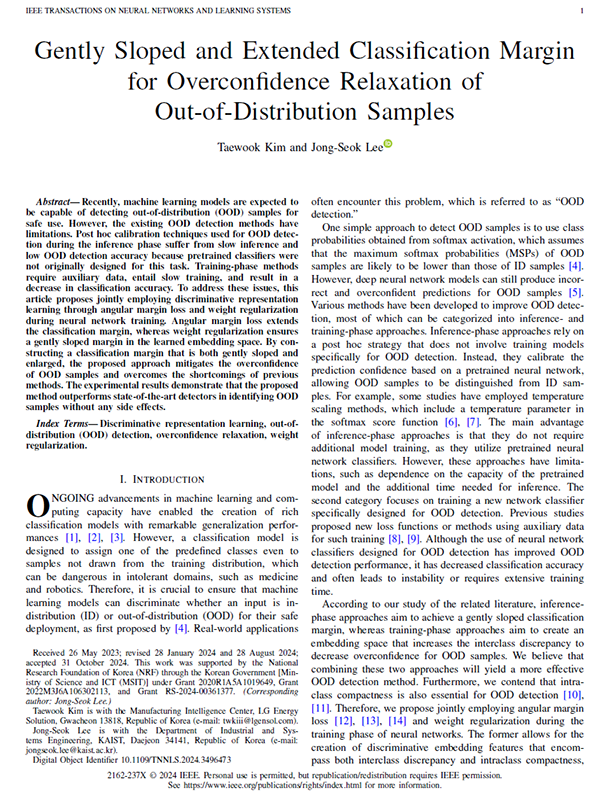
Journal | IEEE Transactions on Neural Networks and Learning Systems (Early Access) |
---|---|
Name | T. Kim and J.-S. Lee |
Year | 2024 |
Recently, machine learning models are expected to be capable of detecting out-of-distribution (OOD) samples for safe use. However, the existing OOD detection methods have limitations. Post hoc calibration techniques used for OOD detection during the inference phase suffer from slow inference and low OOD detection accuracy because pretrained classifiers were not originally designed for this task. Training-phase methods require auxiliary data, entail slow training, and result in a decrease in classification accuracy. To address these issues, this article proposes jointly employing discriminative representation learning through angular margin loss and weight regularization during neural network training. Angular margin loss extends the classification margin, whereas weight regularization ensures a gently sloped margin in the learned embedding space. By constructing a classification margin that is both gently sloped and enlarged, the proposed approach mitigates the overconfidence of OOD samples and overcomes the shortcomings of previous methods. The experimental results demonstrate that the proposed method outperforms state-of-the-art detectors in identifying OOD samples without any side effects.