When costs are unequal and unknown: a subtree grafting approach for unbalanced data classification
페이지 정보

조회 1,472회 작성일 20-12-19 15:30
본문
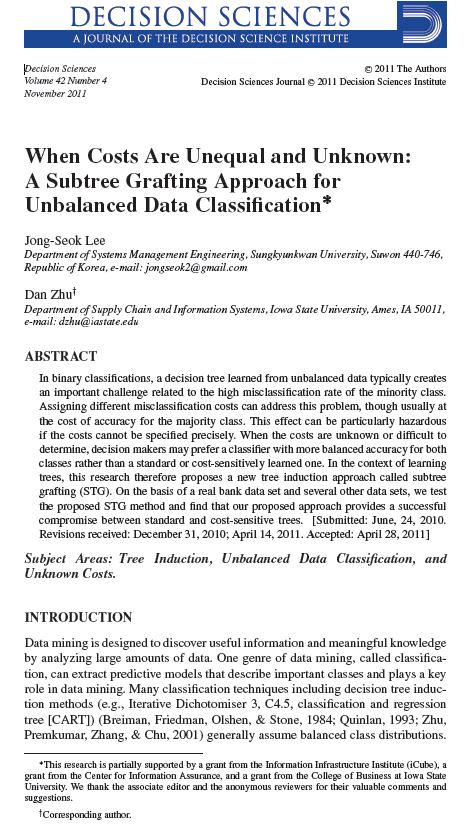
Journal | Decision Sciences, 42(4), 803-829. |
---|---|
Name | Lee, J.-S. and Zhu, D. |
Year | 2011 |
In binary classifications, a decision tree learned from unbalanced data typically creates an important challenge related to the high misclassification rate of the minority class. Assigning different misclassification costs can address this problem, though usually at the cost of accuracy for the majority class. This effect can be particularly hazardous if the costs cannot be specified precisely. When the costs are unknown or difficult to determine, decision makers may prefer a classifier with more balanced accuracy for both classes rather than a standard or cost‐sensitively learned one. In the context of learning trees, this research therefore proposes a new tree induction approach called subtree grafting (STG). On the basis of a real bank data set and several other data sets, we test the proposed STG method and find that our proposed approach provides a successful compromise between standard and cost‐sensitive trees.