Temporal Peak-Management and Clustering System for Sustainable Learning With Application to the Excimer Laser Annealing Process
페이지 정보

조회 682회 작성일 23-03-17 16:34
본문
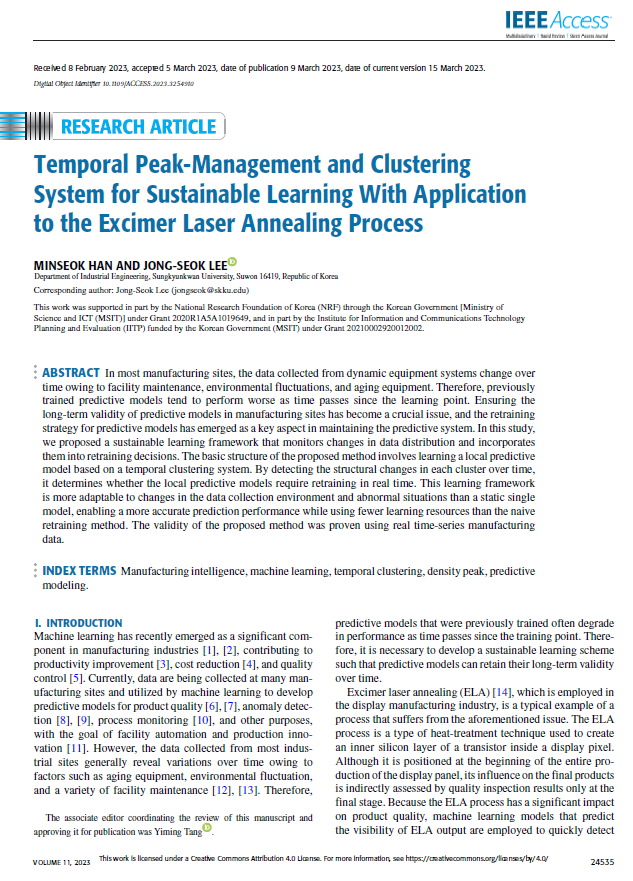
Journal | IEEE Access, 11, 24535-24544 |
---|---|
Name | Han, M. and Lee, J.-S. |
Year | 2023 |
In most manufacturing sites, the data collected from dynamic equipment systems change over time owing to facility maintenance, environmental fluctuations, and aging equipment. Therefore, previously trained predictive models tend to perform worse as time passes since the learning point. Ensuring the long-term validity of predictive models in manufacturing sites has become a crucial issue, and the retraining strategy for predictive models has emerged as a key aspect in maintaining the predictive system. In this study, we proposed a sustainable learning framework that monitors changes in data distribution and incorporates them into retraining decisions. The basic structure of the proposed method involves learning a local predictive model based on a temporal clustering system. By detecting the structural changes in each cluster over time, it determines whether the local predictive models require retraining in real time. This learning framework is more adaptable to changes in the data collection environment and abnormal situations than a static single model, enabling a more accurate prediction performance while using fewer learning resources than the naive retraining method. The validity of the proposed method was proven using real time-series manufacturing data.