Two-way cooperative prediction for collaborative filtering recommendations
페이지 정보

조회 1,550회 작성일 20-12-08 17:23
본문
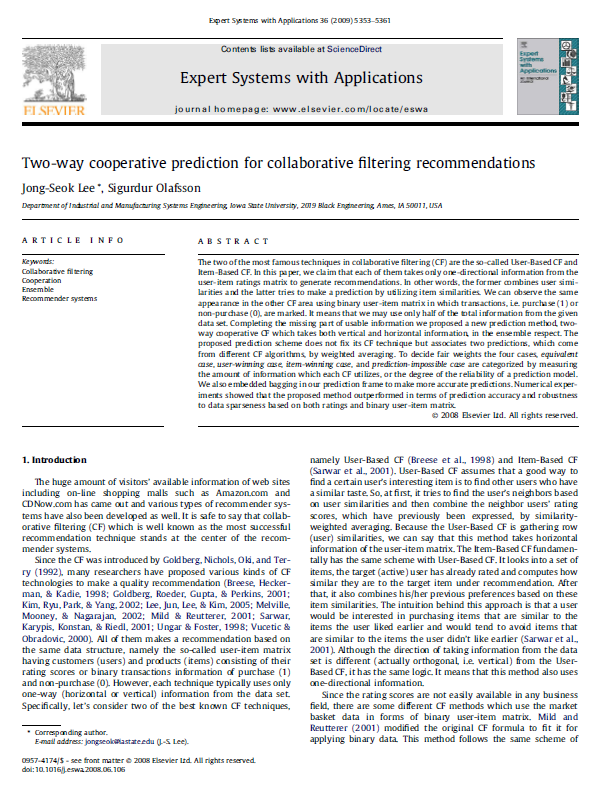
Journal | Expert Systems with Applications, 36(3), 5353-5361. |
---|---|
Name | Lee, J.-S. and Olafsson, S. |
Year | 2009 |
The two of the most famous techniques in collaborative filtering (CF) are the so-called User-Based CF and Item-Based CF. In this paper, we claim that each of them takes only one-directional information from the user-item ratings matrix to generate recommendations. In other words, the former combines user similarities and the latter tries to make a prediction by utilizing item similarities. We can observe the same appearance in the other CF area using binary user-item matrix in which transactions, i.e. purchase (1) or non-purchase (0), are marked. It means that we may use only half of the total information from the given data set. Completing the missing part of usable information we proposed a new prediction method, two-way cooperative CF which takes both vertical and horizontal information, in the ensemble respect. The proposed prediction scheme does not fix its CF technique but associates two predictions, which come from different CF algorithms, by weighted averaging. To decide fair weights the four cases, equivalent case, user-winning case, item-winning case, and prediction-impossible case are categorized by measuring the amount of information which each CF utilizes, or the degree of the reliability of a prediction model. We also embedded bagging in our prediction frame to make more accurate predictions. Numerical experiments showed that the proposed method outperformed in terms of prediction accuracy and robustness to data sparseness based on both ratings and binary user-item matrix.