A precise ranking method for outlier detection
페이지 정보

조회 1,925회 작성일 20-12-19 16:03
본문
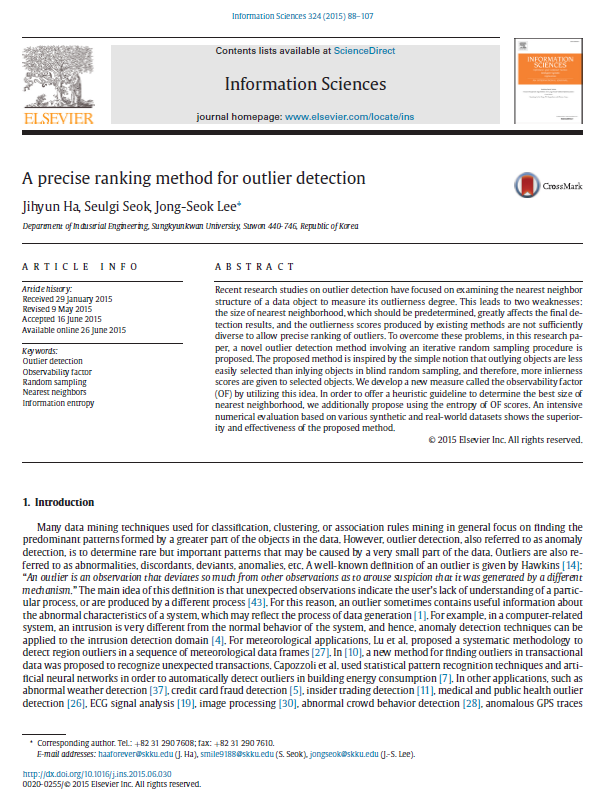
Journal | Information Sciences, 324, 88-107. |
---|---|
Name | Ha, J., Seok, S., and Lee, J.-S. |
Year | 2015 |
Recent research studies on outlier detection have focused on examining the nearest neighbor structure of a data object to measure its outlierness degree. This leads to two weaknesses: the size of nearest neighborhood, which should be predetermined, greatly affects the final detection results, and the outlierness scores produced by existing methods are not sufficiently diverse to allow precise ranking of outliers. To overcome these problems, in this research paper, a novel outlier detection method involving an iterative random sampling procedure is proposed. The proposed method is inspired by the simple notion that outlying objects are less easily selected than inlying objects in blind random sampling, and therefore, more inlierness scores are given to selected objects. We develop a new measure called the observability factor (OF) by utilizing this idea. In order to offer a heuristic guideline to determine the best size of nearest neighborhood, we additionally propose using the entropy of OF scores. An intensive numerical evaluation based on various synthetic and real-world datasets shows the superiority and effectiveness of the proposed method.