Recommendation framework combining user interests with fashion trends in apparel online shopping
페이지 정보

조회 2,031회 작성일 20-12-19 21:52
본문
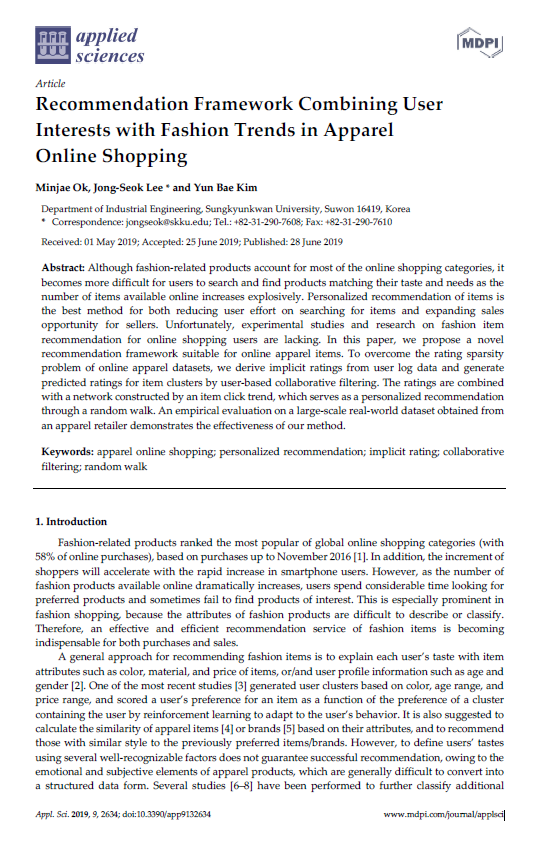
Journal | Applied Sciences, 9(13), 2634. |
---|---|
Name | Ok, M., Lee, J.-S., and Kim, Y. B. |
Year | 2019 |
Although fashion-related products account for most of the online shopping categories, it becomes more difficult for users to search and find products matching their taste and needs as the number of items available online increases explosively. Personalized recommendation of items is the best method for both reducing user effort on searching for items and expanding sales opportunity for sellers. Unfortunately, experimental studies and research on fashion item recommendation for online shopping users are lacking. In this paper, we propose a novel recommendation framework suitable for online apparel items. To overcome the rating sparsity problem of online apparel datasets, we derive implicit ratings from user log data and generate predicted ratings for item clusters by user-based collaborative filtering. The ratings are combined with a network constructed by an item click trend, which serves as a personalized recommendation through a random walk. An empirical evaluation on a large-scale real-world dataset obtained from an apparel retailer demonstrates the effectiveness of our method.