Convergence analysis of connection center evolution and faster clustering
페이지 정보

조회 1,475회 작성일 22-03-15 12:55
본문
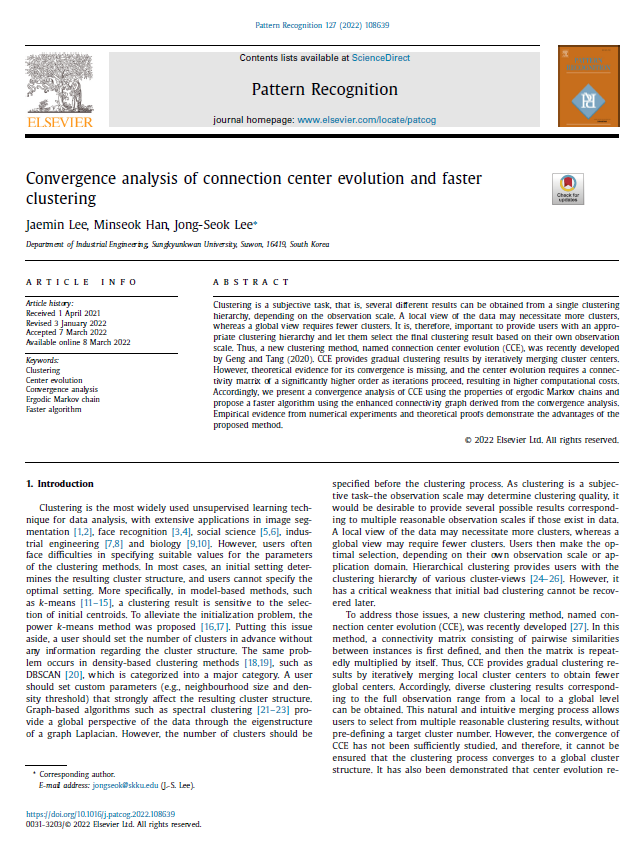
Journal | Pattern Recognition, 127, 108639 |
---|---|
Name | Lee, J., Han, M., and Lee, J.-S. |
Year | 2022 |
Clustering is a subjective task, that is, several different results can be obtained from a single clustering hierarchy, depending on the observation scale. A local view of the data may necessitate more clusters, whereas a global view requires fewer clusters. It is, therefore, important to provide users with an appropriate clustering hierarchy and let them select the final clustering result based on their own observation scale. Thus, a new clustering method, named connection center evolution (CCE), was recently developed by Geng and Tang (2020). CCE provides gradual clustering results by iteratively merging cluster centers. However, theoretical evidence for its convergence is missing, and the center evolution requires a connectivity matrix of a significantly higher order as iterations proceed, resulting in higher computational costs. Accordingly, we present a convergence analysis of CCE using the properties of ergodic Markov chains and propose a faster algorithm using the enhanced connectivity graph derived from the convergence analysis. Empirical evidence from numerical experiments and theoretical proofs demonstrate the advantages of the proposed method.