Learning From Imbalanced Data Using Triplet Adversarial Samples
페이지 정보

조회 687회 작성일 23-04-04 15:02
본문
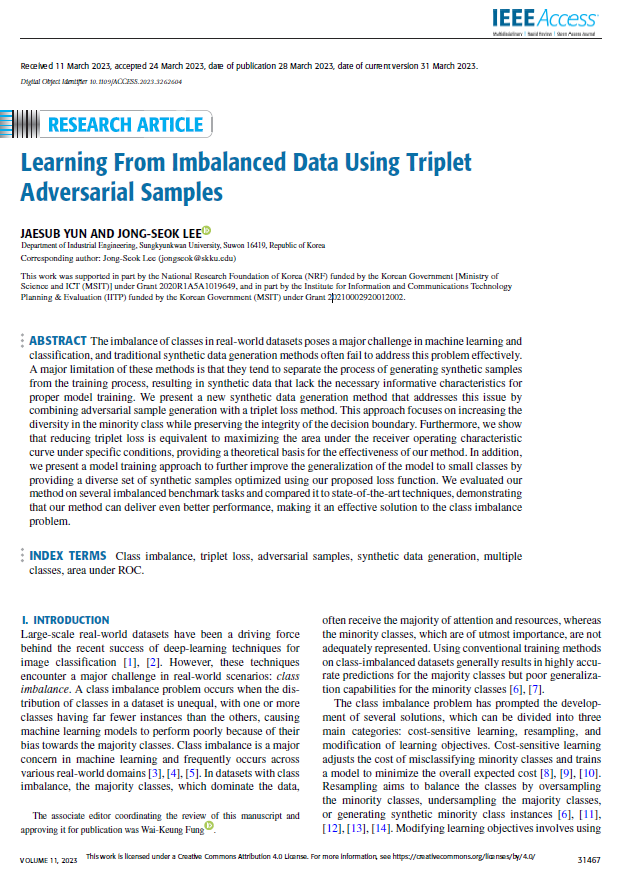
Journal | IEEE Access, 11, 31467-31478 |
---|---|
Name | Yun, J. and Lee, J.-S. |
Year | 2023 |
The imbalance of classes in real-world datasets poses a major challenge in machine learning and classification, and traditional synthetic data generation methods often fail to address this problem effectively. A major limitation of these methods is that they tend to separate the process of generating synthetic samples from the training process, resulting in synthetic data that lack the necessary informative characteristics for proper model training. We present a new synthetic data generation method that addresses this issue by combining adversarial sample generation with a triplet loss method. This approach focuses on increasing the diversity in the minority class while preserving the integrity of the decision boundary. Furthermore, we show that reducing triplet loss is equivalent to maximizing the area under the receiver operating characteristic curve under specific conditions, providing a theoretical basis for the effectiveness of our method. In addition, we present a model training approach to further improve the generalization of the model to small classes by providing a diverse set of synthetic samples optimized using our proposed loss function. We evaluated our method on several imbalanced benchmark tasks and compared it to state-of-the-art techniques, demonstrating that our method can deliver even better performance, making it an effective solution to the class imbalance problem.